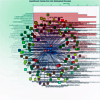
|
|
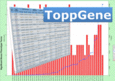
|
- Name: ToppGene
- Purpose: works by generating a representative profile of the training genes using as many as 17 features and identifies over-representative terms from the training genes. This forms the first step and is done by using ToppFun component. The test set genes are compared to this representative profile of the training set or the overrepresented terms from the training genes.
- Access and Help: same as above
- Reference: Chen et al., 2007 (Citing articles via Google Scholar).
|
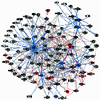
|
- Name: ToppNet
- Purpose: Gene prioritization based on protein–protein interaction network (PPIN) analyses. Based on the observation that biological networks share many properties with Web and social networks, ToppNet uses extended versions of three algorithms from White and Smyth —PageRank with Priors, HITS with Priors and K-step Markov — to prioritize disease candidate genes by estimating their relative importance in the PPIN to the disease-related genes.
- Access and Help: same as above
- Reference: Chen etal., 2009 (Citing articles via Google Scholar).
|
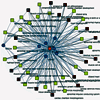
|
- Name: ToppGeNet
- Purpose: differs from ToppGene and ToppNet in that the test set is derived from the protein interactome. In other words, for a training set of known disease genes, the test set is generated by mining the protein interactome and compiling the genes either directly or indirectly interacting (based on user input) with the training set. The interactome-based test set genes can be prioritized using either a functional annotation-based method (ToppGene) or PPIN-based method (ToppNet).
- Access and Help: same as above
- Reference: Chen etal., 2009 (Citing articles via Google Scholar)
|
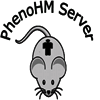
|
- Name: PhenoHM
- Purpose: Human–mouse comparative phenome–genome server that facilitates cross-species identification of genes associated with orthologous phenotypes. By cross-mapping mouse–human phenotype terms, extracting implicated genes and extrapolating phenotype-gene associations between species PhenoHM enables rapid identification of genes that trigger similar outcomes in human and mouse.
- Reference: Sardana et al., 2010 (Citing articles via Google Scholar)
|
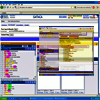
|
- Name: GATACA
- Purpose: GATACA or Gene(tic) Associations To Anatomy and Clinical Abnormalities is a disease-centered knowledgebase that enables biomedical researchers to explore, analyze, and hypothesize genetic pathways, networks and processes responsible for disease.
- Access: http://gataca.cchmc.org
- Status: Ongoing
|
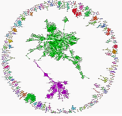
|
|
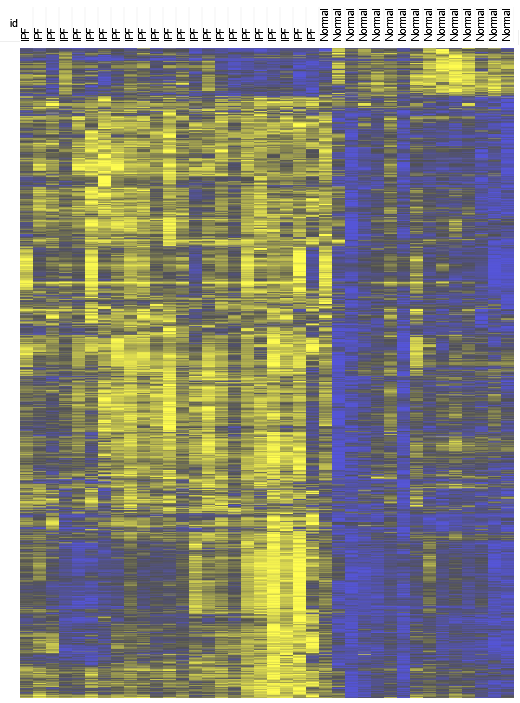
|
- Name: IPF Database
- Purpose: Compilation of differentially expressed genes in IPF from different published studies.
- Status: Ongoing
|
|